Space and Time, a Web3 Startup, Aims to Enhance Data Transparency in the AI Sector
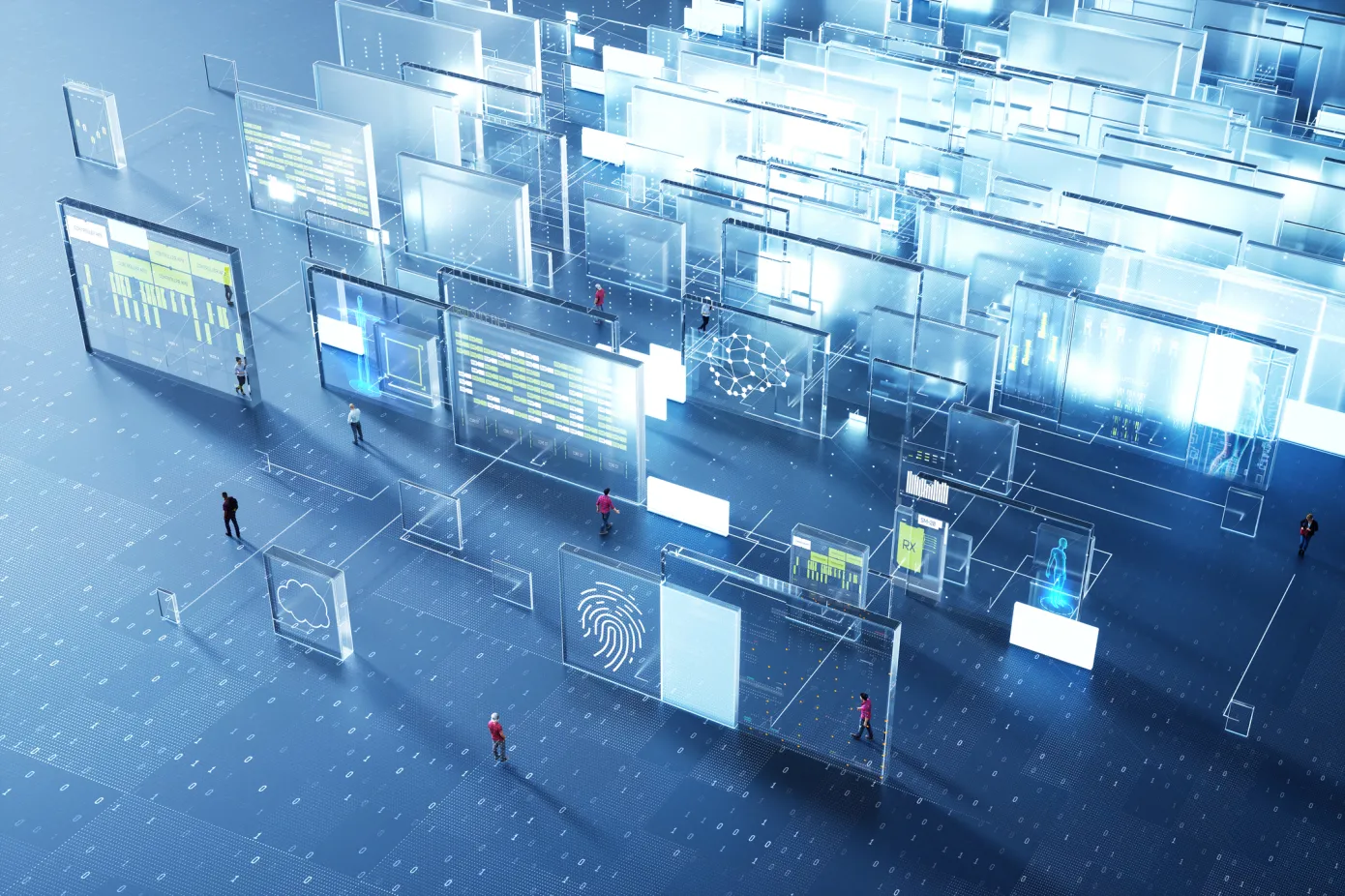
Dykstra highlights a critical issue in the realm of data manipulation, drawing parallels between the collapse of FTX and the broader challenge of trust in financial institutions and other industries. He emphasizes the need for solutions that ensure the integrity and accuracy of data, particularly in contexts where there are strong incentives for bad actors to manipulate information.
One such solution Dykstra advocates for is the use of zero-knowledge proofs (ZK proofs), a cryptographic technique that enables the verification of data without revealing the underlying information. ZK proofs operate on the principle of allowing a prover to confirm the truth of a statement to a verifier without disclosing any additional details beyond the statement’s correctness.
In the context of financial data or sensitive information, ZK proofs offer a means to verify the accuracy of records or transactions without exposing the data itself, thereby mitigating the risk of manipulation or fraud. Dykstra sees ZK proofs as a fundamental tool for ensuring the integrity of data across various domains, including finance, blockchain, and artificial intelligence (AI).
Space and Time, Dykstra’s venture, aims to provide a verifiable computing layer for Web3 by indexing data from both off-chain and on-chain sources, leveraging ZK proofs to enhance data integrity. While the startup initially focuses on blockchain data, Dykstra envisions its application expanding to other industries where data verification is crucial.
However, Dykstra also raises concerns about the verifiability of AI-generated data, particularly with large language models (LLMs). He underscores the importance of developing ZK proofs tailored for machine learning to address the risk of tampering or manipulation by model operators.
While solutions for ZK proofs in the context of LLMs are in development, Dykstra acknowledges the challenges and time required to implement such mechanisms effectively. Nonetheless, he emphasizes the urgency of ensuring the integrity of AI-generated data to maintain trust and transparency in AI systems.