The cost of AI training data is so high that only Big Tech companies can afford it
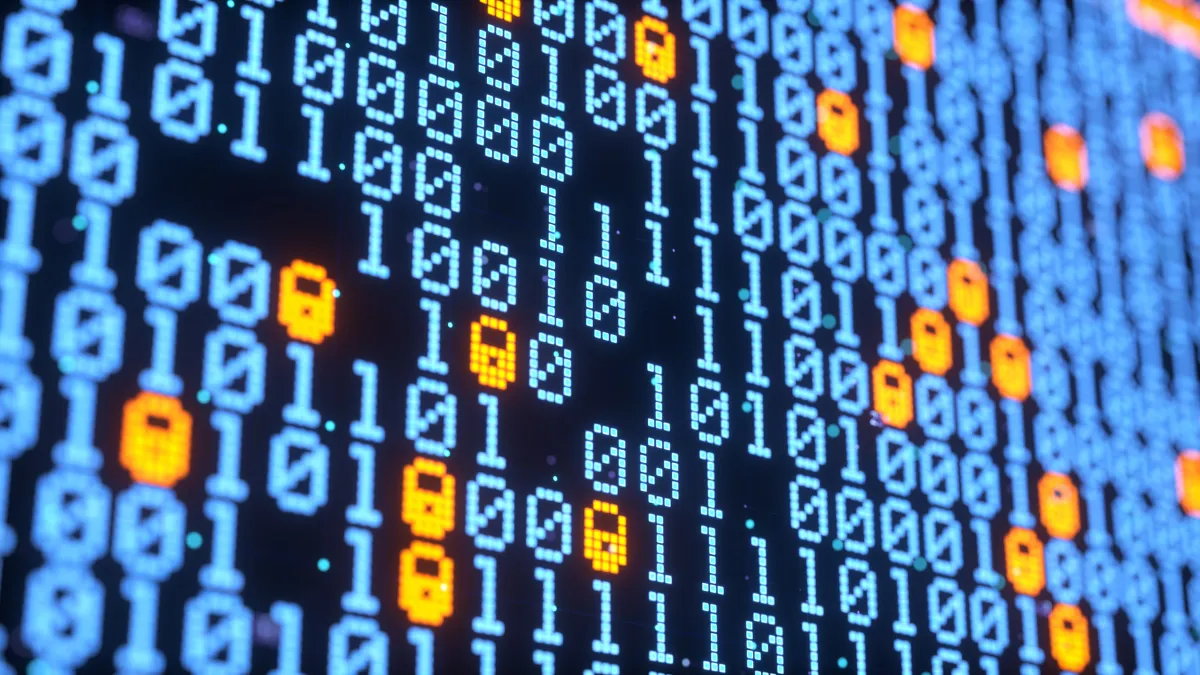
Data is undeniably at the core of today’s advanced AI systems, but the escalating costs of high-quality datasets are making them increasingly inaccessible to all but the wealthiest tech companies.
In a personal blog post last year, James Betker, a researcher at OpenAI, emphasized the critical role of training data in the development of generative AI models. Betker argued that the key to the sophistication and capabilities of AI systems lies not in their design or architecture but in the datasets on which they are trained.
“Trained on the same dataset for long enough, pretty much every model converges to the same point,” Betker wrote.
So, is Betker correct? Is training data the primary factor determining what an AI model can achieve, whether it’s answering a question, drawing human hands, or generating a realistic cityscape?
This assertion holds substantial weight. The quality and diversity of the data used to train AI models are crucial because they directly influence the model’s ability to generalize from the training data to new, unseen scenarios. High-quality data helps models learn more nuanced and accurate representations of the world, improving their performance in various tasks.
However, it’s important to note that while training data is a significant determinant, other factors also contribute to the effectiveness of an AI model. These include:
1. **Model Architecture**: The design of the neural network and its layers can significantly affect the model’s ability to learn from data and perform complex tasks.
2. **Training Algorithms**: The methods and algorithms used to train the model can impact its efficiency and accuracy. Innovations in training techniques often lead to significant improvements in model performance.
3. **Computational Resources**: The amount of computational power available can determine how thoroughly a model can be trained on a given dataset. More compute allows for training on larger datasets and more complex models.
4. **Hyperparameter Tuning**: The process of adjusting the parameters that control the training process can optimize performance and improve results.
While Betker’s assertion that “pretty much every model converges to the same point” when trained on the same dataset for long enough highlights the importance of data, it’s a simplification. The interplay between data, model architecture, training algorithms, computational resources, and hyperparameter tuning collectively determines the final performance of an AI system.
In conclusion, training data is indeed a fundamental component of AI development, but it works in concert with other critical factors to shape the capabilities of AI models. The rising costs of high-quality datasets pose a significant barrier, emphasizing the need for more accessible data-sharing frameworks and collaborative efforts to democratize AI advancements.