Diffusion transformers serve as the cornerstone of OpenAI’s Sora, poised to revolutionize GenAI
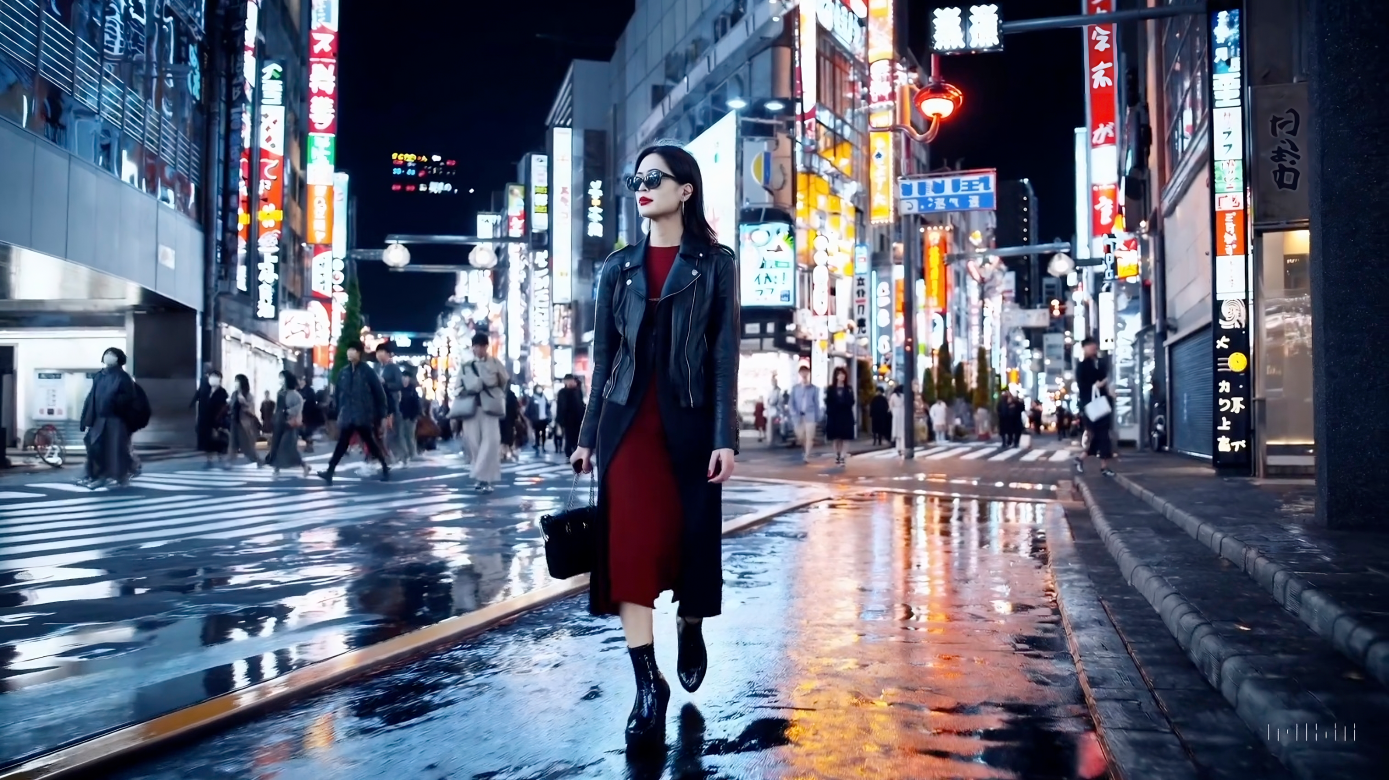
The diffusion transformer, developed by Saining Xie and William Peebles, combines two concepts in machine learning—diffusion and the transformer—to create a powerful AI model architecture. This innovation has significant implications for the field of Generative AI (GenAI), enabling models to scale up beyond previous limits.
Saining Xie, a computer science professor at NYU, initiated the research project in June 2022, during which he collaborated with William Peebles, then interning at Meta’s AI research lab and now co-leading the Sora project at OpenAI. The diffusion transformer has since become a foundational technology for various AI-powered media generators, including OpenAI’s Sora and Stability AI’s Stable Diffusion 3.0.
Diffusion, a process commonly used in modern AI media generators like OpenAI’s DALL-E 3, involves the generation of images, videos, speech, music, 3D meshes, and artwork. By integrating diffusion with the transformer architecture, the diffusion transformer extends the capabilities of AI models, allowing them to produce content with unprecedented realism, complexity, and scale.
The diffusion process involves gradually adding noise to a piece of media, such as an image, until it becomes unrecognizable. This noisy media is then used to train a diffusion model, which learns to gradually remove the noise, ultimately reaching the target output media (e.g., a clean image).
Traditionally, diffusion models employ a “backbone” called a U-Net, which is responsible for estimating and removing the noise. However, U-Nets can be complex and may slow down the diffusion pipeline due to their specially-designed modules.
Transformers offer an alternative to U-Nets in diffusion models. By replacing U-Nets with transformers, efficiency and performance can be improved. Transformers excel at processing sequential data, making them well-suited for tasks like image generation and noise removal in the diffusion process. This substitution enhances the overall speed and effectiveness of the diffusion model.