Despite its impressive predictive capabilities, the research team underscores that the AI tool should be viewed as a starting point for future advancements rather than a definitive solution.
Tina Eliassi-Rad, a professor at Northeastern University in the US, emphasizes caution in employing this tool for real-time predictions concerning individuals. “Even though we’re using prediction to evaluate how good these models are, the tool shouldn’t be used for prediction on real people,” she asserts, clarifying that the tool’s predictions are rooted in a specific dataset pertaining to a particular population.
With an intent to infuse a human-centric approach into AI development, the team sought involvement from social scientists during the tool’s creation. By doing so, they aimed to ensure that amidst the colossal dataset on which the tool was trained, the human aspect remained central and not overshadowed.
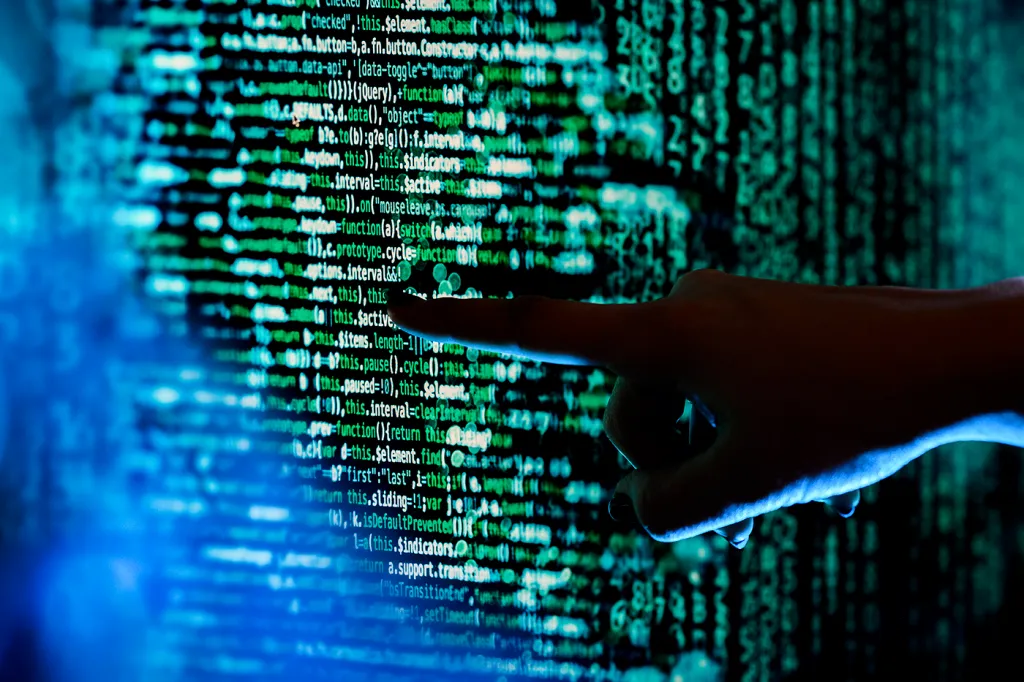
Sune Lehmann, an author of the study published in the journal Nature Computational Science, highlights the comprehensive nature of life2vec, stating, “This model offers a much more comprehensive reflection of the world as it is lived by human beings than many other models.”
At the core of life2vec lies the extensive dataset used to train the model. The researchers crafted extended sequences of recurring life events from this dataset, applying a transformer model approach similar to training language models like LLMs but adapted for the representation of a human life through event sequences.
Describing the model’s operation, Lehmann, a professor at the Technical University of Denmark, likens the entirety of a human life to “a giant long sentence” composed of various life occurrences.
Life2vec employs the acquired knowledge from millions of life event sequences to generate vector representations within embedding spaces. These spaces facilitate the categorization and linkage of life events such as income, education, or health factors.
The resulting embedding spaces serve as the foundation for the model’s predictions, especially in scenarios like predicting the probability of mortality.
Lehmann elucidates on the model’s visualization, likening it to “a long cylinder” encompassing low to high probabilities of death. The model’s accuracy is validated by correlating high probabilities of death with actual instances of mortality, while areas with low probabilities might feature unpredictable events like fatal accidents, Lehmann concludes.