Introducing Google Genie: A Game-Changer in AI-Generated 2D Platformer Games
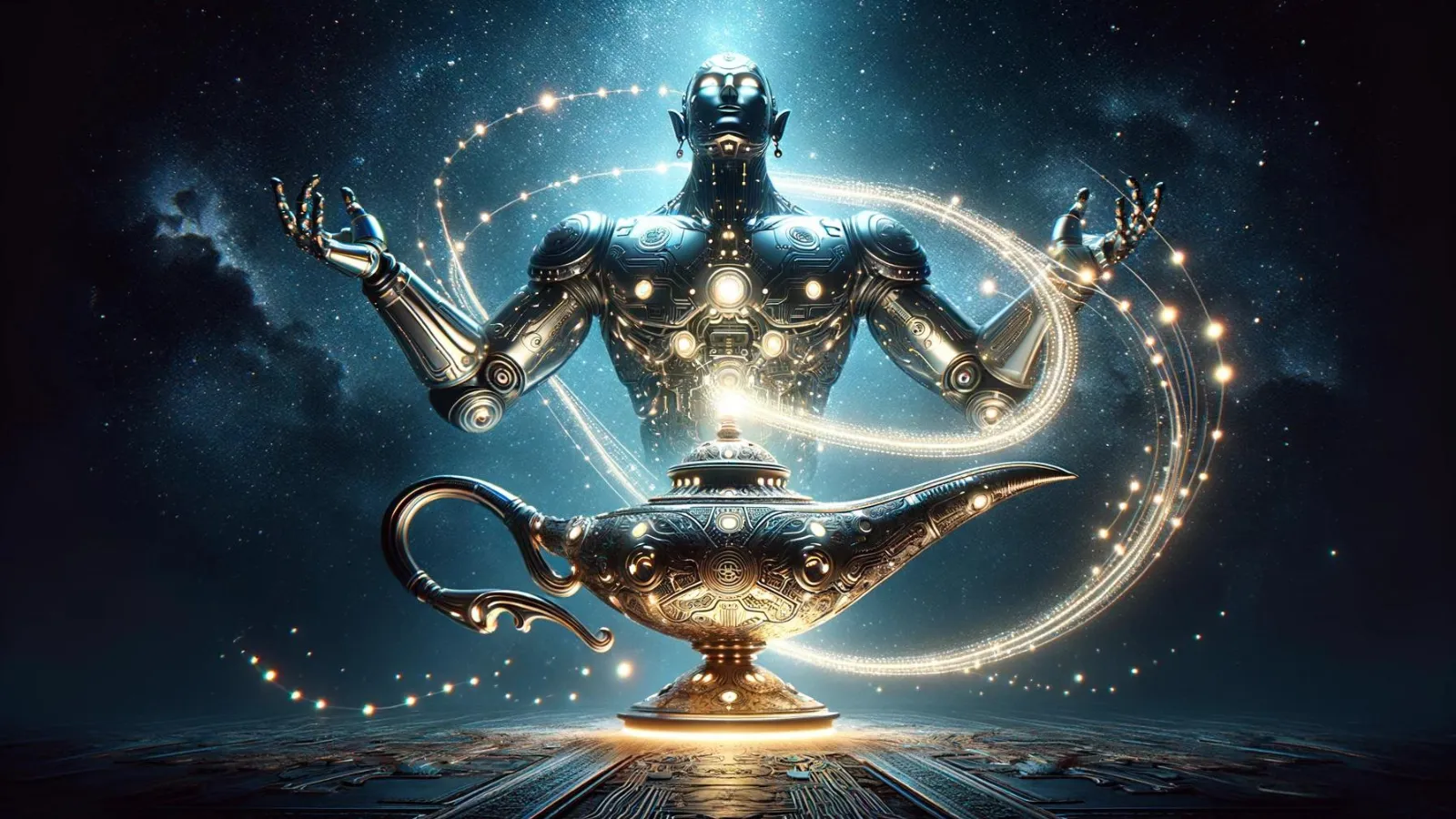
Unlocking Creativity: How Google’s Genie AI Model Learnt from 2D Platformer Videos
In a groundbreaking move, Google has unveiled Genie, an innovative generative AI model designed to revolutionize the creation of 2D platformer video games. Genie represents a significant leap forward in the realm of AI-driven game development, offering the ability to generate an infinite array of dynamic and engaging game levels.
Developed by the team at Google DeepMind, Genie leverages advanced predictive analysis techniques to craft immersive gaming experiences, complete with controllable characters and dynamic environmental elements. This cutting-edge technology promises to redefine the boundaries of creativity in the gaming industry.
The development of Genie marks a notable advancement in the field of AI-driven content creation. Unlike previous models, which were limited in scope and functionality, Genie represents a more sophisticated approach to generative AI. By training exclusively on vast datasets of unsupervised video game footage, Genie has acquired a deep understanding of the underlying mechanics and dynamics of 2D platformer games. This comprehensive knowledge base enables Genie to generate highly realistic and diverse game levels, tailored to the specific preferences and requirements of developers and players alike.
While Genie is currently in the research phase and not yet available to the public, its potential implications for the gaming industry are immense. With its ability to seamlessly generate playable game levels from image prompts, Genie opens up a world of possibilities for developers seeking to create compelling and engaging gaming experiences. As the technology continues to evolve, it is likely that Genie will play a pivotal role in shaping the future of game development, empowering developers to unleash their creativity and imagination like never before.
To simplify, the spatiotemporal video tokenizer takes video game footage, breaks it down into smaller chunks of datasets, known as tokens, that can be consumed by the foundation model. Spatiotemporal explains that the data is broken down both in time and space (For example, a video was broken down into 2-second clips, but each frame was also broken down into multiple pieces).
The autoregressive dynamic model comes next. Autoregressive models essentially predict the future based on how something has performed in the past, and a dynamic model is responsible for understanding how things change and move over time. So this part is where the predictive analysis begins. The final component is the latent action model. This is where the AI understands how the playable character moves and traverses in the video game world.
“Genie’s learned latent action space is not just diverse and consistent, but also interpretable. After a few turns, humans generally figure out a mapping to semantically meaningful actions (like going left, right, jumping etc.),” said Rocktäschel. This part is important because it highlights that the main problem this AI model solves is not just generating 2D video game levels, but also understanding how basic movements occur, and how that information can be used to navigate real-world terrains.