Large Language Models Empower Home Robots to Self-Recover from Errors Without Human Intervention
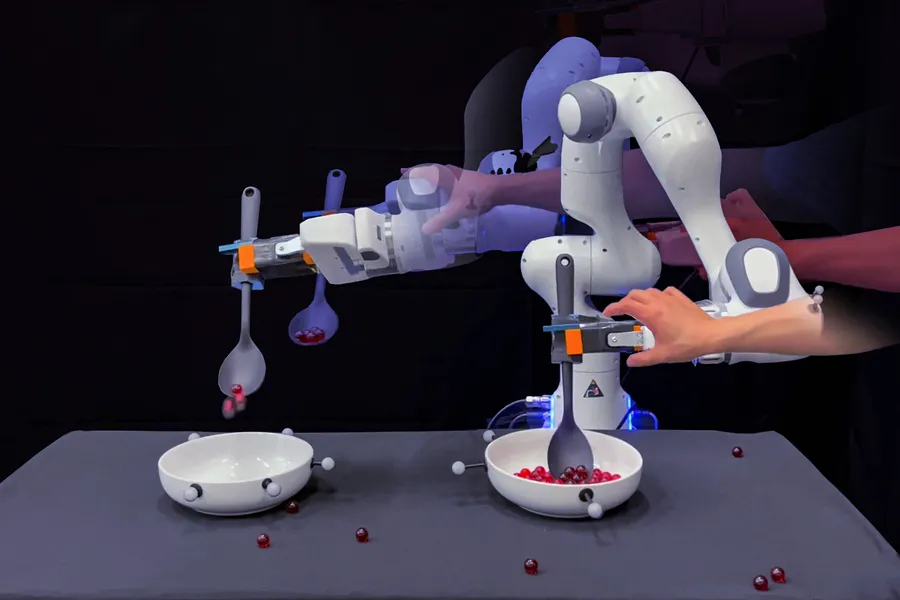
The use of large language models (LLMs) in the robotics space, as demonstrated by new research from MIT, aims to address the challenge of correcting mistakes made by home robots in unstructured environments. Traditionally, when a robot encounters issues, it exhausts its pre-programmed options before requiring human intervention, which can be particularly challenging in home settings where environmental variations are common.
The study, set to be presented at the International Conference on Learning Representations (ICLR), introduces a method to bring “common sense” into the process of correcting mistakes. It leverages the fact that robots are excellent mimics but may struggle to adjust to unexpected situations without explicit programming.
One key aspect of the research is breaking demonstrations into smaller subsets, rather than treating them as continuous actions. This approach allows the robot to learn from observed actions in a more granular manner, enabling it to adapt to small environmental variations more effectively.
LLMs play a crucial role in this process by eliminating the need for manual labeling and assignment of subactions by the programmer. Instead, the model learns from the observed demonstrations and can generate appropriate responses to unforeseen circumstances based on the context provided.
By integrating LLMs into home robotics, researchers aim to enhance the robots’ ability to navigate and operate in dynamic environments, ultimately improving their practicality and usability for consumers. This approach represents a step forward in addressing the challenges that have hindered the success of home robots beyond devices like Roomba.