Study Identifies Five New Brain Aging Patterns from Analysis of 50,000 Brain Scans
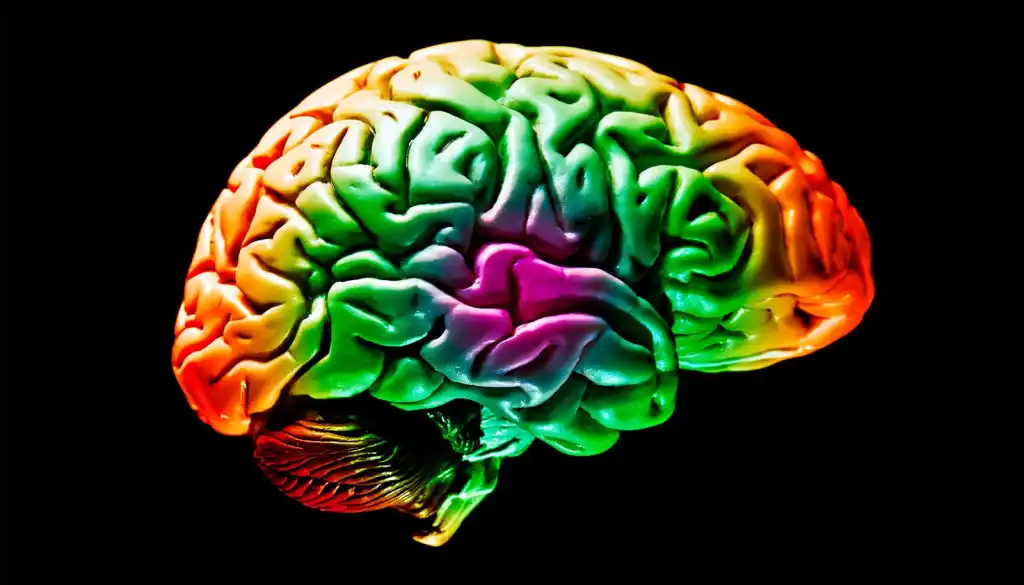
Study Links New Brain Aging Patterns to Lifestyle Factors Like Smoking and Alcohol Consumption
A groundbreaking study has unveiled new patterns of brain aging that could signal neurodegenerative diseases or brain atrophy, following an extensive analysis of nearly 50,000 brain scans. The research, conducted by a team of scientists using advanced machine-learning techniques, identifies up to five distinct patterns of brain deterioration. These findings offer fresh insights into how the brain ages and deteriorates over time, potentially enhancing early detection methods for conditions such as dementia and Parkinson’s disease. By pinpointing these patterns at an earlier stage, the study could pave the way for more effective predictive tools and preventive strategies.
Published in Nature Medicine on August 15, 2024, the study utilized a sophisticated deep-learning algorithm known as Semi-Supervised Representation Learning via GAN (Surreal-GAN). GAN, or generative adversarial networks, is a class of algorithms that pits two neural networks against each other to improve their performance. In this case, Surreal-GAN was trained on MRI scans from over 10,000 individuals, including both healthy subjects and those experiencing cognitive decline. This robust dataset provided a comprehensive foundation for the algorithm to learn and identify subtle variations in brain structure.
The Surreal-GAN algorithm excelled in detecting minute changes in brain anatomy that are often too subtle for manual observation. By analyzing the MRI scans, the algorithm was able to recognize recurring features and develop a model that identifies patterns of anatomical changes. These patterns, which are indicative of brain degeneration, were classified into five distinct types. This capability to detect fine-grained alterations could significantly improve our understanding of the early stages of brain diseases.
The identification of these five brain aging patterns offers a promising avenue for early diagnosis and intervention. The patterns could serve as biomarkers for neurodegenerative conditions, potentially allowing for earlier and more accurate detection. Early identification of such patterns could enable timely medical interventions, lifestyle modifications, or targeted therapies that may help slow down or mitigate the progression of brain diseases.
Moreover, the study’s findings also highlight the role of lifestyle factors in brain aging. The researchers found correlations between the identified brain aging patterns and lifestyle factors such as smoking and alcohol consumption. These associations suggest that lifestyle choices may influence brain health and aging processes, providing valuable information for developing preventive measures and public health strategies aimed at reducing the risk of neurodegenerative diseases.
The implications of this research are substantial, not only for the field of neurology but also for the broader understanding of brain health. By leveraging advanced machine-learning techniques to analyze a vast amount of data, the study has opened new possibilities for the early detection and management of brain aging and related conditions. As research progresses, these findings could lead to the development of more precise diagnostic tools and tailored interventions that address the underlying patterns of brain degeneration, ultimately improving outcomes for individuals at risk of neurodegenerative diseases.