Apple Collaborates with Nvidia to Enhance AI Model Performance and Speed
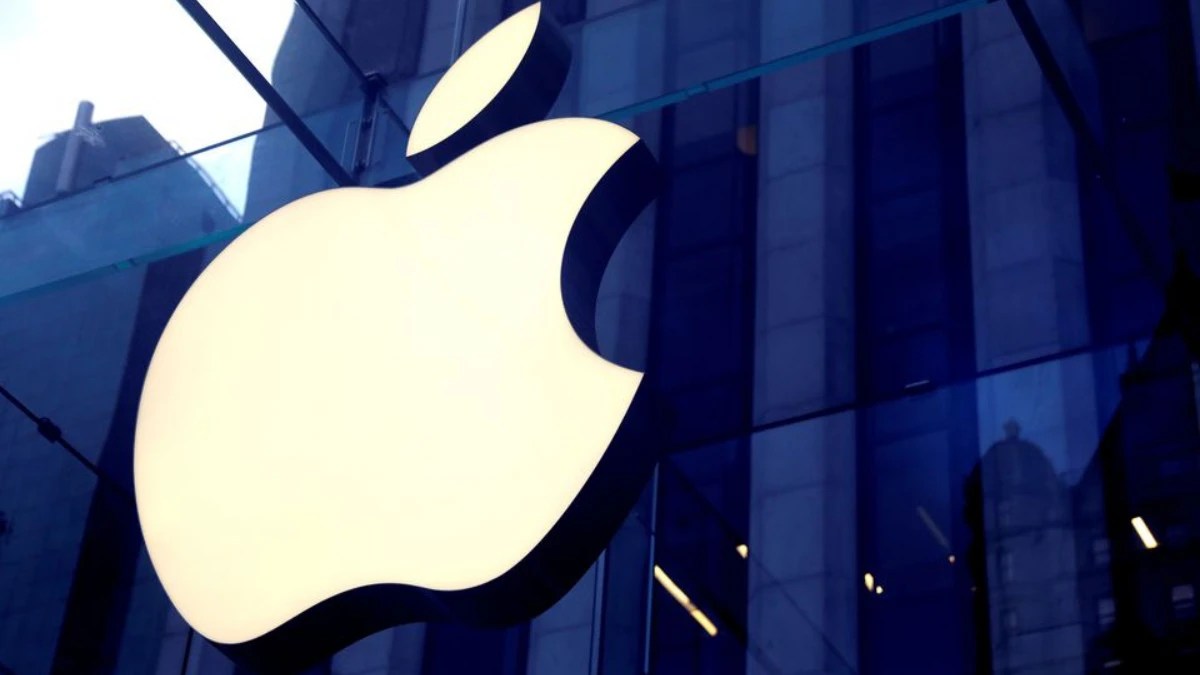
Apple has announced a new partnership with Nvidia to enhance the performance and speed of artificial intelligence (AI) models. The collaboration is focused on accelerating inference processes, aiming to boost both efficiency and latency in large language models (LLMs). Apple revealed that its researchers have been working extensively on this challenge, leveraging Nvidia’s platform to explore whether improvements can be achieved in both areas simultaneously. The effort incorporates Apple’s Recurrent Drafter (ReDrafter) technique, which was detailed in a research paper earlier this year, in combination with Nvidia’s powerful TensorRT-LLM framework designed for inference acceleration.
In a blog post outlining the details of the partnership, Apple emphasized the importance of refining AI model inference processes to make them faster and more efficient. The company’s engineers have been tackling the complex issue of improving LLM performance while ensuring that latency—the time it takes for a model to respond—is kept to a minimum. By fine-tuning both elements, Apple aims to optimize AI workflows and make them more reliable and faster in real-world applications.
For context, inference in machine learning refers to the phase where a trained model processes input data and generates predictions or decisions. This step is crucial as it allows AI models to provide valuable insights or actions based on the data they are given. It is in this phase that the raw input is translated into meaningful output, such as text generation, image classification, or decision-making, depending on the nature of the model.
Through this collaboration, Apple and Nvidia hope to set a new benchmark for AI model performance. By improving the efficiency of large language models and reducing latency, they aim to accelerate the deployment of AI technologies across various industries. This partnership represents a significant step forward in refining the computational capabilities needed for next-generation AI applications, benefiting everything from virtual assistants to more complex, data-driven processes.