“Embarrassing and wrong”: Google acknowledges loss of control over image-generating AI
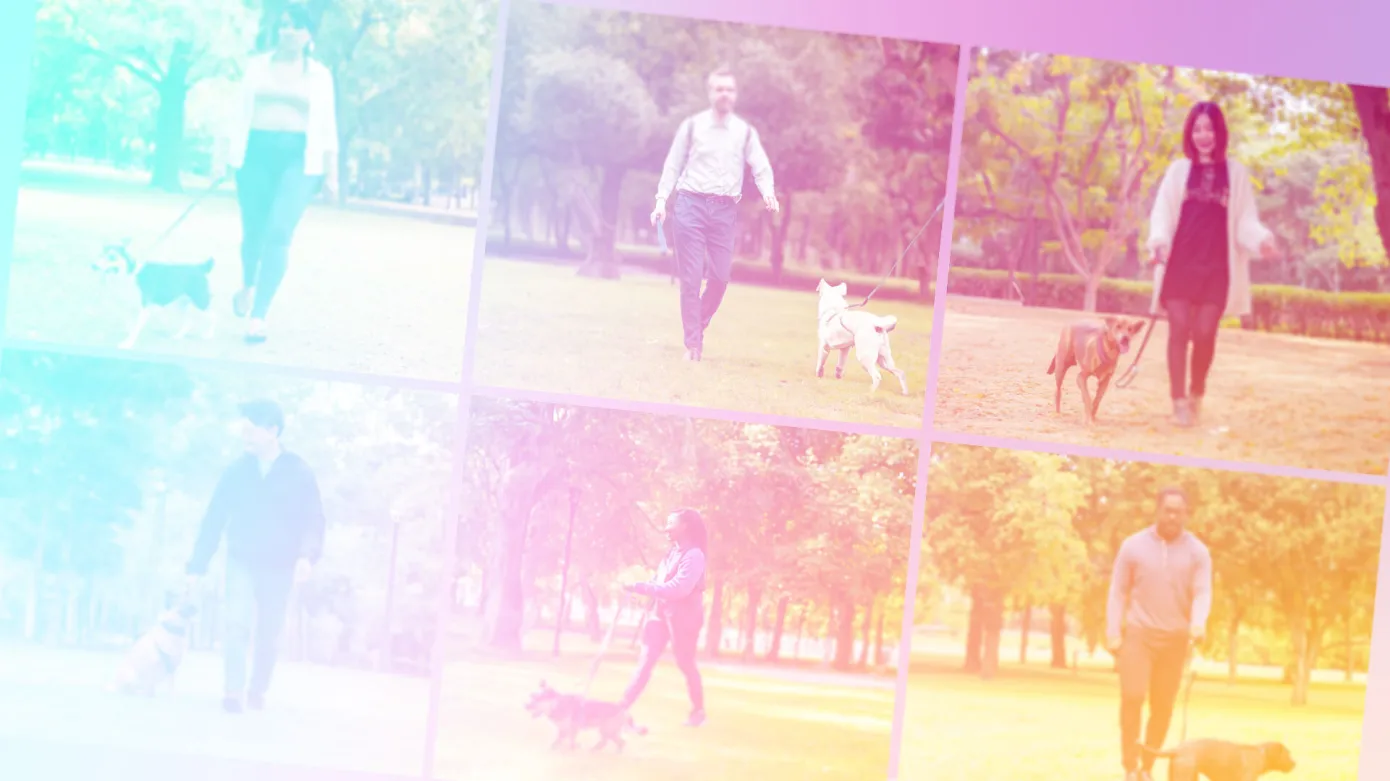
Google has issued an apology (or something very close to it) for yet another embarrassing AI blunder this week. The issue involved an image-generating model, part of Google’s flagship conversational AI platform called Gemini, which injected diversity into images with a comical disregard for historical accuracy. While the underlying problem is understandable, Google seemed to blame the model itself for “becoming” oversensitive, which drew criticism since the model was developed by humans.
When users requested imagery of certain historical circumstances or figures, the AI produced laughable results. For example, the Founding Fathers, known to be white slave owners, were depicted as a multicultural group, including people of color.
This embarrassing and easily reproducible issue was quickly ridiculed by commentators online. It also became entangled in the ongoing discourse surrounding diversity, equity, and inclusion, which is currently facing significant scrutiny. Pundits seized upon the incident as evidence of what they perceive as a “woke” ideology infiltrating the already liberal tech sector.
Amidst the uproar, some conspicuously concerned citizens decried the situation as “DEI gone mad,” attributing it to the current political climate in America under President Biden. Google was labeled an “ideological echo chamber,” accused of being a proxy for left-leaning ideologies. However, even individuals on the left expressed unease with the situation.
However, as those familiar with the technology can attest, Google’s explanation in its apology-adjacent post sheds light on the issue. The problem stemmed from a reasonable workaround for systemic bias in training data.
Imagine you’re using Gemini to create a marketing campaign and request 10 images of “a person walking a dog in a park.” Without specifying the type of person, dog, or park, the generative model will default to what it’s most familiar with. And often, this familiarity is shaped by the training data, which may contain various biases.
In many image collections, such as stock imagery or rights-free photography, white people are overrepresented. Consequently, if not specified, the model defaults to generating images with white individuals.